Revolutionizing agricultural practices, real-time field analysis using drone imagery is rapidly transforming how farmers monitor and manage their crops. This innovative approach allows for immediate assessment of field conditions, enabling timely interventions for optimal yield and resource management. From detecting crop stress to optimizing irrigation, drones equipped with advanced sensors provide valuable data, enabling a more precise and efficient agricultural process.
The technology offers a wealth of data points, including multispectral, hyperspectral, and thermal imagery, providing a comprehensive view of the field. This data, processed in real-time, offers crucial insights into crop health, nutrient levels, and potential issues, enabling farmers to respond promptly to challenges and maximize productivity.
Introduction to Drone Imagery in Agriculture
Unmanned aerial vehicles (UAVs), commonly known as drones, are rapidly transforming agricultural practices. Their ability to capture high-resolution imagery from the sky provides unprecedented insights into crop health, soil conditions, and overall farm management. This real-time data analysis facilitates precise interventions, maximizing yields, and minimizing resource consumption. The use of drones in agriculture is no longer a futuristic concept but a practical tool enhancing efficiency and sustainability in farming.Drones offer significant advantages over traditional methods of field assessment.
The speed and efficiency of aerial surveys allow for comprehensive coverage of large tracts of land in a fraction of the time required for ground-based inspections. This rapid assessment is critical for timely interventions, such as detecting disease outbreaks or identifying areas requiring irrigation adjustments. This capability enables farmers to react swiftly to emerging issues, preserving crop health and maximizing yields.
Drone Imagery Types for Agricultural Applications
Various types of drone imagery are crucial for different agricultural needs. Multispectral imagery captures data across a broader range of wavelengths beyond the visible spectrum. This provides information about vegetation health and stress, which is valuable for identifying nutrient deficiencies or pest infestations. Hyperspectral imagery goes further, recording data across numerous narrow spectral bands. This detailed data allows for a more precise analysis of plant physiology and stress, enabling targeted treatments.
Thermal imagery detects variations in surface temperature, which is vital for identifying water stress in crops or detecting heat-related issues. This data is essential for optimizing irrigation strategies and preventing crop damage.
Technical Aspects of Data Acquisition
The acquisition of drone imagery involves several technical considerations. Calibration of the drone’s sensors is crucial to ensure accurate data interpretation. Precise flight paths and overlapping imagery are essential for creating high-resolution mosaics of the field. Advanced image processing techniques are often employed to analyze the collected data, which can involve image enhancement, filtering, and classification to isolate specific features like crop type or disease.
These techniques allow for objective analysis of vast datasets, facilitating quick decision-making.
Drone Contributions to Agricultural Practices
Drones are revolutionizing agricultural practices, particularly in precision farming. The ability to map crop health and identify areas needing attention allows for targeted application of fertilizers, pesticides, and water. This precision reduces waste and minimizes environmental impact. Drone imagery enables the creation of detailed maps of field conditions, allowing for variable rate application of inputs, which is essential for optimizing resource use and profitability.
This data-driven approach reduces overapplication, optimizing resource use and enhancing sustainability.
Precision Farming with Drone Data
The detailed insights provided by drone imagery allow for more precise interventions in farming. This is especially valuable in precision farming where the goal is to optimize resource use and maximize yields. Farmers can identify specific areas of the field needing targeted interventions. For example, they can apply fertilizers or pesticides only to those areas where they are needed, minimizing waste and environmental impact.
This approach leads to more efficient resource utilization, enhanced profitability, and a more sustainable agricultural system.
Different Types of Drones for Agricultural Use
Drone Type | Key Specifications |
---|---|
Lightweight, fixed-wing drones | Excellent for large-area coverage; typically faster than multirotor drones; often equipped with high-resolution cameras |
Multirotor drones (quadcopters) | Versatile; suitable for various terrains; often equipped with multispectral or hyperspectral sensors; relatively easy to maneuver |
Specialized agricultural drones | Equipped with specific sensors for agricultural applications, like advanced cameras and thermal imaging; designed for durability and reliability in harsh environments; capable of long flight times |
Data from drone imagery can lead to significant improvements in agricultural practices, from precise fertilizer application to early detection of crop stress.
Real-Time Data Processing and Analysis
Rapid advancements in drone technology are revolutionizing agricultural practices, enabling real-time data acquisition and analysis. This allows farmers to make informed decisions on the fly, responding swiftly to emerging issues like crop stress or disease outbreaks. The ability to process drone imagery in real-time offers a significant advantage over traditional methods, which often involve delayed data collection and analysis.Real-time processing involves a streamlined workflow, beginning with the drone’s capture of high-resolution images and concluding with immediate feedback for the farmer.
This immediacy empowers informed, agile responses to crop conditions, optimizing resource allocation and minimizing potential losses. The processing pipeline involves multiple stages, each crucial for accurate and actionable results.
Image Preprocessing Techniques
Image preprocessing is a critical initial step for accurate real-time analysis. These steps ensure the data is clean and suitable for subsequent analysis. This involves reducing noise and artifacts that can skew results, as well as correcting for geometric distortions introduced during image acquisition.
- Noise Reduction: Noise in drone imagery, often caused by atmospheric conditions or sensor limitations, can obscure subtle features. Advanced filtering techniques, like median filtering or wavelet denoising, effectively remove noise while preserving important details.
- Geometric Correction: Drone imagery frequently suffers from geometric distortions due to variations in flight paths and terrain. Geometric correction rectifies these distortions, aligning the image with a reference system. This ensures accurate spatial analysis and enables comparison across different images.
Extracting Relevant Information
The core of real-time analysis lies in extracting meaningful data from the imagery. This process identifies crucial parameters that indicate crop health and potential issues.
- Vegetation Indices: Vegetation indices (VIs) are quantitative measures derived from spectral reflectance data. These indices, such as Normalized Difference Vegetation Index (NDVI), are valuable indicators of vegetation health, photosynthetic activity, and stress levels. Higher NDVI values generally indicate healthier crops.
- Crop Health Assessment: Analyzing VIs and other spectral characteristics allows for the assessment of overall crop health. Variations in these indices can highlight areas of stress, nutrient deficiencies, or disease. By monitoring these indicators, farmers can proactively address issues before they impact yield.
Identifying Crop Stress Indicators
Identifying crop stress early is crucial for mitigating potential yield losses. Real-time analysis enables farmers to react swiftly to emerging problems.
- Identifying Stress: Analyzing spectral data allows the identification of specific stress indicators, such as variations in chlorophyll content, water stress, or nutrient deficiencies. Specific spectral signatures are linked to particular stress types, enabling precise identification and targeted interventions.
- Analyzing Stress Indicators: The analysis focuses on identifying subtle variations in the spectral signature that indicate the presence of stress. This enables timely intervention and potential mitigation strategies.
Software Tools and Platforms
Various software platforms facilitate real-time image analysis, offering intuitive interfaces and powerful processing capabilities.
- Software Options: Specialized software packages and cloud-based platforms provide tools for image processing, analysis, and visualization. These tools often include pre-built algorithms for vegetation index calculation and crop health assessment.
- Real-Time Processing Capabilities: Real-time processing capabilities depend on the chosen platform and the complexity of the analysis. Cloud-based platforms are particularly well-suited for handling large volumes of data and providing immediate results.
Comparison of Real-Time Image Processing Techniques
Technique | Strengths | Weaknesses |
---|---|---|
Median Filtering | Effective at reducing noise, preserves important details | May blur sharp edges, less effective with complex noise patterns |
Wavelet Denoising | Adaptive to various noise types, maintains image details | Computationally more intensive than median filtering |
NDVI Calculation | Simple, widely used for vegetation assessment | Sensitive to atmospheric conditions, may not capture subtle stress indicators |
Applications of Real-Time Field Analysis
Real-time analysis of drone imagery in agriculture is revolutionizing farming practices, enabling farmers to make data-driven decisions with unprecedented speed and precision. This dynamic approach to field assessment allows for immediate adjustments to irrigation, fertilization, disease management, and pest control, ultimately leading to optimized yields and resource utilization.
Optimizing Irrigation Scheduling
Real-time soil moisture monitoring via drone imagery allows farmers to precisely assess water needs across their fields. By identifying areas experiencing varying levels of dryness, farmers can implement targeted irrigation strategies. This avoids overwatering in some sections while ensuring adequate hydration in others. This dynamic approach reduces water waste and enhances crop health. For example, a farmer using real-time data might find that one section of their field requires irrigation while another section has sufficient moisture, enabling precise scheduling and resource allocation.
Managing Fertilizer Application
Real-time analysis of nutrient levels in the soil and plant health enables targeted fertilizer application. Drones equipped with multispectral cameras can capture detailed information about nutrient deficiencies in specific areas. This allows for precise fertilizer application, minimizing waste and environmental impact. Farmers can apply fertilizers only where they are needed, thus improving crop yields and reducing costs associated with unnecessary fertilizer use.
Identifying and Treating Crop Diseases
Real-time image analysis can rapidly identify the presence and spread of crop diseases. By detecting subtle changes in leaf color, texture, and other visual cues, the system can flag areas needing attention. This early detection enables timely interventions, preventing the spread of the disease and minimizing crop loss. For instance, early detection of fungal diseases can be achieved through real-time analysis, enabling immediate fungicide application to a specific area, preventing widespread infection.
Improving Pest Management Strategies
Real-time monitoring of pest populations through drone imagery allows for proactive pest management. By identifying areas with high pest concentrations, farmers can deploy targeted pest control measures, minimizing the use of pesticides and protecting beneficial insects. This proactive approach improves crop health and reduces environmental risks associated with broad-spectrum pesticide use. Using real-time data, a farmer can quickly identify a pest infestation in one part of the field, allowing for a localized and timely response to the issue.
Yield Prediction and Forecasting
Real-time analysis can provide valuable insights into potential crop yields. By analyzing factors like plant health, soil conditions, and environmental data, the system can create dynamic yield predictions. This information enables farmers to make informed decisions about harvesting schedules and optimize resource allocation. Using historical data combined with real-time conditions, the system can provide estimates of yield, allowing farmers to plan for harvest accordingly.
Precision Livestock Management
Real-time analysis can enhance precision livestock management by tracking animal health and behavior. Drone imagery combined with sensor data can monitor animal grazing patterns, body condition scores, and other relevant indicators. This information aids in identifying sick or stressed animals, enabling timely interventions. Real-time monitoring can track animal movement patterns, which helps manage grazing areas effectively.
Applications Across Agricultural Sectors
Agricultural Sector | Real-Time Field Analysis Applications |
---|---|
Crop Production | Irrigation scheduling, fertilizer application, disease and pest management, yield prediction |
Livestock Management | Animal health monitoring, grazing pattern analysis |
Challenges and Future Directions
Real-time analysis of drone imagery for agricultural applications holds immense promise, but significant hurdles remain. The integration of this technology into existing farming practices faces obstacles ranging from technical limitations to concerns about data security and privacy. Overcoming these challenges is crucial for realizing the full potential of this innovative approach to precision agriculture.
Technical Challenges in Real-Time Analysis
Real-time processing of drone imagery requires substantial computational power and sophisticated algorithms. Current technologies struggle to analyze large datasets quickly enough for immediate actionable insights in the field. The high volume of data generated by high-resolution sensors and the need for near-instantaneous processing present a considerable challenge. Processing delays can prevent farmers from making timely decisions, potentially impacting crop yields and overall efficiency.
Limitations of Current Technologies in Real-Time Field Analysis
Current image processing algorithms, while improving, often lack the accuracy and robustness needed for complex agricultural scenarios. For instance, distinguishing between different crop types, identifying subtle signs of stress or disease, or accurately measuring soil moisture in real-time remains a significant limitation. Additionally, the variability in lighting conditions, weather patterns, and terrain features can negatively impact the quality and reliability of the analyzed data.
Furthermore, the need for stable and reliable internet connectivity for data transmission and processing in remote locations is a crucial concern.
Challenges in Integrating Drone Data with Existing Agricultural Management Systems
Integrating drone imagery data into existing agricultural management software and databases is not straightforward. The heterogeneity of data formats and the lack of standardized protocols create compatibility issues. Current systems may not be equipped to handle the volume and velocity of drone-generated data. This lack of integration can lead to data silos and hinder the effective use of the valuable information collected.
Data standardization and interoperability between different platforms and systems are key to addressing this challenge.
Potential of Emerging Technologies to Enhance Real-Time Analysis
Emerging technologies such as cloud computing, artificial intelligence (AI), and machine learning (ML) offer promising avenues for enhancing real-time analysis. Cloud platforms can provide the necessary computing power to process large datasets quickly. AI and ML algorithms can be trained to identify patterns and anomalies in the imagery, allowing for more accurate and automated analysis. These technologies can significantly improve the speed and accuracy of data processing, enabling real-time decision-making.
For example, AI-powered image analysis can identify crop stress earlier, enabling proactive intervention.
Data Security and Privacy Concerns
Data security and privacy are critical considerations for drone imagery in agriculture. The sensitive nature of the data collected, including location information and crop health details, requires robust security measures to protect it from unauthorized access. Privacy regulations and ethical considerations need to be carefully addressed to ensure that farmers’ data remains confidential and that their rights are respected.
Examine how drone-based crop monitoring for pest detection and control can boost performance in your area.
Implementing secure data transmission protocols and encryption techniques is crucial to mitigate risks. For instance, anonymizing or aggregating data can help maintain privacy without compromising the value of the information.
Future Research Directions in Real-Time Analysis
Future research should focus on developing more sophisticated and accurate real-time analysis methods. This includes exploring novel image processing techniques, refining AI and ML algorithms, and improving data integration with existing agricultural management systems. Research into developing more robust and adaptable algorithms for various environmental conditions is essential. Furthermore, investigating the potential of sensor fusion, combining drone imagery with other data sources like weather forecasts and soil sensors, could lead to more comprehensive and accurate analyses.
Comparison of Real-Time Data Analysis Techniques
Technique | Benefits | Drawbacks |
---|---|---|
Cloud-based processing | Scalability, cost-effectiveness, access to powerful computing resources | Dependence on reliable internet connectivity, potential latency issues |
AI/ML-based analysis | Automated pattern recognition, improved accuracy, potential for early detection of anomalies | Algorithm training requirements, potential for bias in algorithms, data privacy concerns |
Sensor fusion | Enhanced data accuracy and comprehensiveness, ability to integrate multiple data sources | Complexity in data integration, potential for increased computational load |
Case Studies and Examples
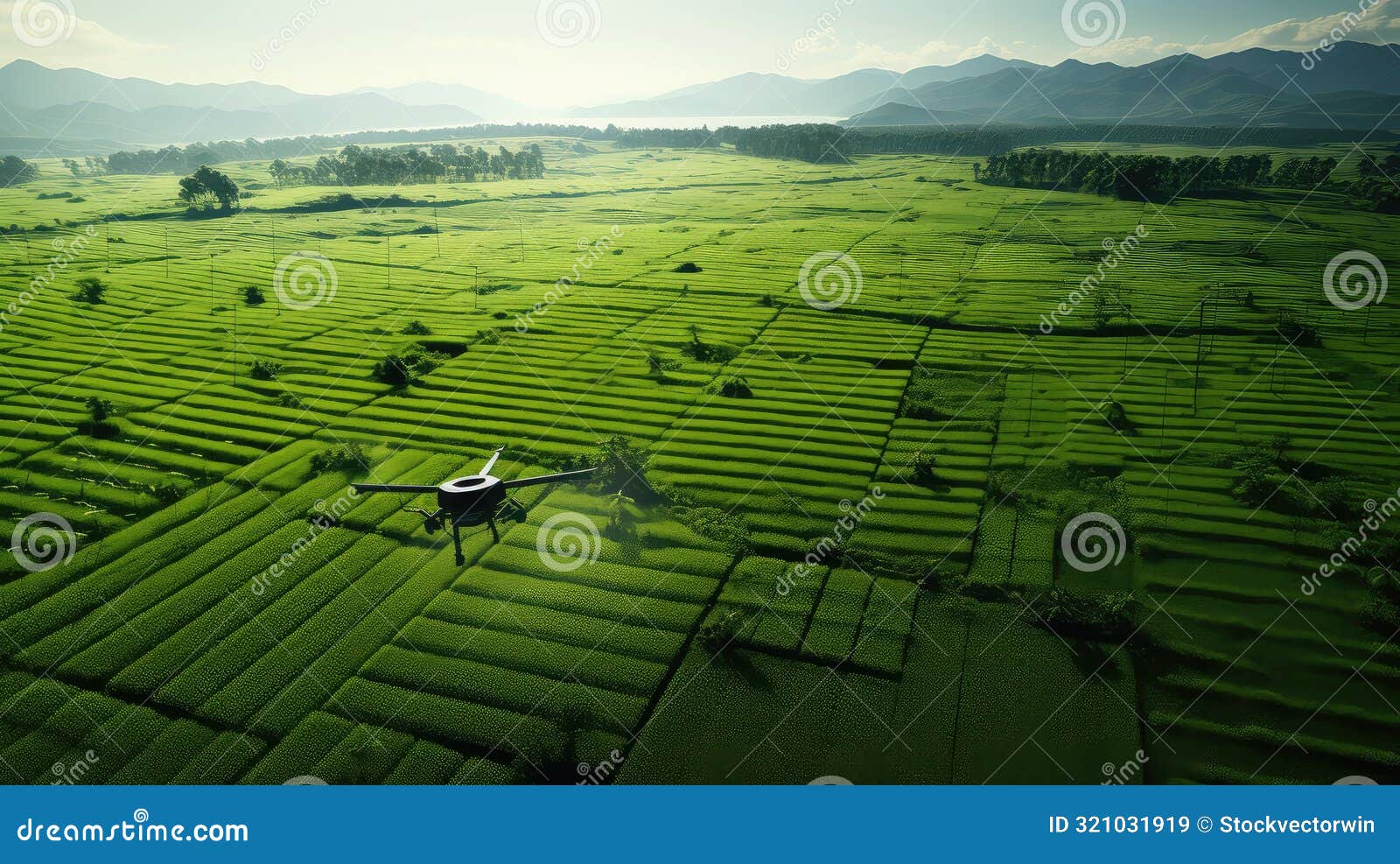
Real-time drone imagery analysis is rapidly transforming agricultural practices, offering farmers unprecedented insights into their fields. This real-time feedback loop, enabled by advanced processing techniques, allows for immediate adjustments to irrigation, fertilization, and pest control, ultimately boosting productivity and profitability. The ability to monitor crop health and identify issues early can significantly reduce losses and improve resource management.This section presents successful case studies illustrating the tangible impact of real-time field analysis on various agricultural settings, demonstrating how drone imagery integration can optimize farm management and promote sustainable practices.
Successful Applications in Vineyards
Real-time analysis of vineyard health is proving invaluable for yield optimization and disease prevention. By identifying stressed vines early, farmers can proactively intervene with targeted irrigation or nutrient applications. This immediate response reduces crop loss and enhances the quality of grapes, ultimately translating into higher market value. For instance, a vineyard in California utilized real-time drone data to detect early signs of powdery mildew.
This allowed for a timely application of fungicides, preventing the disease from spreading and minimizing yield loss.
Applications in Orchards
Drone imagery enables orchards to monitor fruit development, identify pest infestations, and assess the overall health of trees. Real-time data analysis can pinpoint areas needing extra attention, enabling timely interventions to maintain fruit quality and size. One orchard in Washington State used real-time analysis to detect early signs of fruit drop, which resulted in the implementation of targeted treatments to increase fruit set and overall yield.
The ability to identify stressed or diseased trees quickly enabled the orchard to take proactive steps to maintain tree health, reducing the need for costly interventions later in the season.
Real-Time Analysis in Crop Fields
Real-time analysis of crop fields allows for optimized irrigation and fertilization strategies. By pinpointing areas needing water or nutrients, farmers can avoid over-application, conserving water resources and minimizing environmental impact. A corn farm in Iowa used real-time drone data to assess moisture levels in different parts of the field. This data was integrated into the irrigation system, ensuring efficient water use and preventing crop stress.
The result was significant water savings, reduced fertilizer costs, and increased crop yields.
Integration with Farm Management Software
Integrating drone imagery with farm management software creates a seamless workflow for data analysis and decision-making. This allows farmers to access real-time insights directly within their existing software, streamlining operations. For example, a farm management software platform can be configured to automatically receive drone imagery data. This data is then processed and analyzed, providing the farmer with real-time reports on crop health, nutrient deficiencies, or potential pest infestations.
These insights can be directly linked to tasks within the software, such as scheduling irrigation or fertilization, creating a comprehensive and integrated farm management system.
Table: Key Findings from Selected Case Studies
Case Study | Agricultural Setting | Impact on Productivity | Impact on Profitability | Sustainable Practices |
---|---|---|---|---|
California Vineyard | Vineyard | Reduced crop loss, improved grape quality | Increased market value, higher revenue | Efficient use of fungicides, reduced disease spread |
Washington Orchard | Orchard | Increased fruit set, improved fruit quality | Higher yields, enhanced fruit value | Targeted treatments, reduced environmental impact |
Iowa Corn Farm | Crop Field | Optimized water use, reduced fertilizer costs | Increased yields, cost savings | Conserved water resources, minimized environmental impact |
Contribution to Sustainable Agricultural Practices
Real-time analysis using drone imagery contributes significantly to sustainable agricultural practices. By enabling precise and timely interventions, farmers can minimize resource use, reducing environmental impact while maintaining high yields. Targeted application of water, fertilizers, and pesticides avoids unnecessary resource consumption, promoting environmental stewardship and responsible agricultural practices.
Epilogue
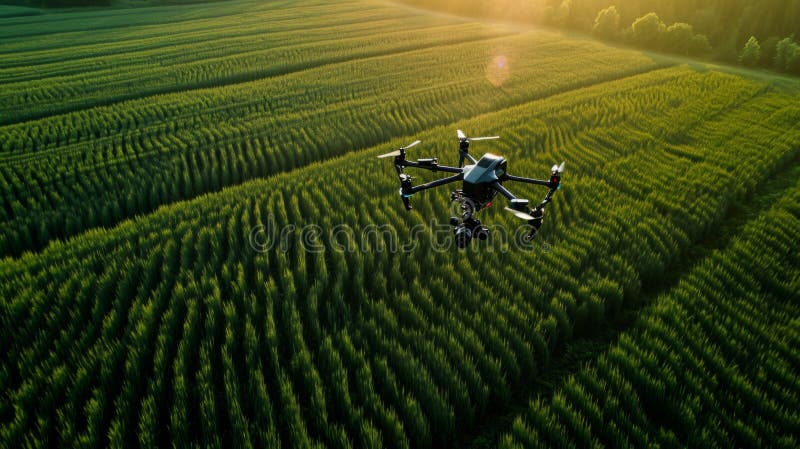
In conclusion, real-time field analysis using drone imagery promises a future of more sustainable and productive agriculture. By providing immediate insights into crop health and potential issues, this technology streamlines decision-making, optimizes resource allocation, and enhances overall farm efficiency. Further advancements in data processing and integration with existing farm management systems will undoubtedly drive even greater adoption and impact across various agricultural sectors.