Drone-aided soil analysis is revolutionizing agricultural practices, offering a precise and efficient approach to targeted nutrient management. Traditional methods often struggle with the variability of soil composition across a field, leading to uneven nutrient application and wasted resources. This new technology promises to address these limitations, enabling farmers to optimize nutrient delivery, minimize environmental impact, and boost crop yields.
The technology leverages advanced sensing capabilities integrated into drones to create detailed maps of soil nutrient content. This data-driven approach allows for the creation of customized fertilization plans, targeting specific areas with precisely the right amount of nutrients. The process is highly efficient, requiring less labor and significantly reducing the environmental footprint of fertilizer application.
Introduction to Drone-Aided Soil Analysis
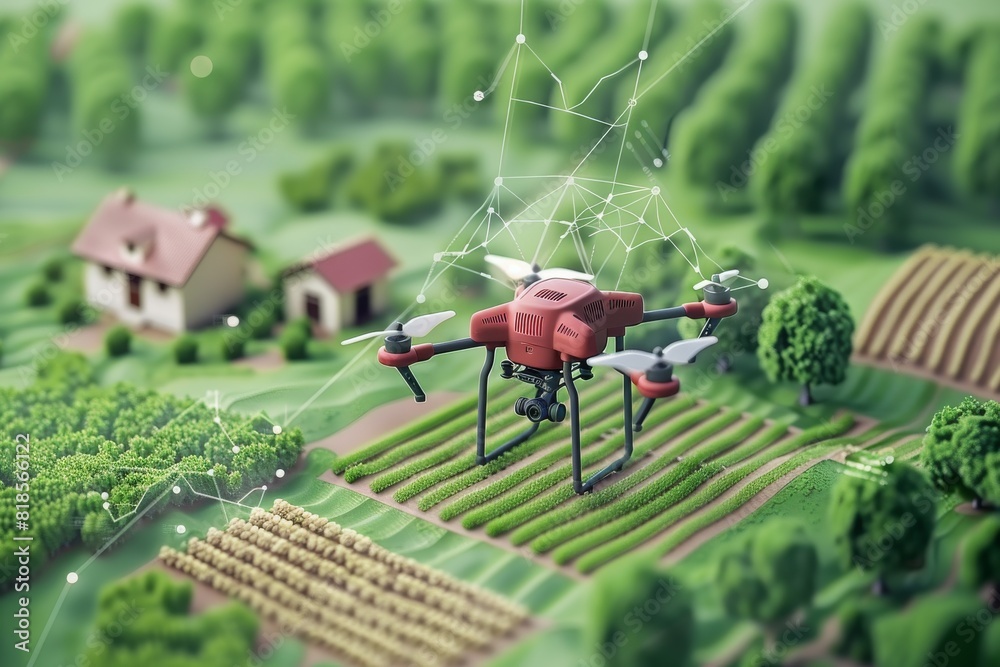
Precision agriculture is rapidly transforming how farmers manage their crops, and a key component of this transformation is targeted nutrient management. Optimal soil nutrient levels are crucial for healthy plant growth, maximizing yields, and minimizing environmental impact. Proper nutrient management ensures crops receive the exact amount of nutrients they need, reducing waste and promoting sustainable agricultural practices. This targeted approach minimizes the use of fertilizers and pesticides, protecting water resources and biodiversity.Traditional methods of soil sampling often struggle to capture the variability within a field.
This heterogeneity in soil composition across even small areas means that a single sample may not accurately reflect the needs of the entire field. This can lead to either over- or under-application of fertilizers, resulting in inefficiencies and environmental concerns. Consequently, there’s a growing need for more sophisticated and precise methods for assessing soil nutrient levels.Drones are emerging as a powerful tool in this regard.
Their ability to capture high-resolution data over large areas, combined with advanced image processing techniques, allows for a much more detailed and comprehensive understanding of soil conditions. This precision translates into more effective fertilizer application, reducing costs and environmental impact. The integration of drone technology with other precision agriculture tools further enhances its efficacy in optimizing crop yields.
Limitations of Traditional Soil Sampling Methods
Traditional soil sampling methods often struggle to capture the variability within a field, leading to inaccurate assessments of nutrient levels. The process is time-consuming and labor-intensive, and may not accurately represent the entire field’s diversity. Traditional methods may not capture subtle variations in soil properties, impacting the effectiveness of targeted nutrient management strategies. This limitation can lead to over- or under-application of fertilizers, resulting in economic and environmental inefficiencies.
Obtain direct knowledge about the efficiency of cost-benefit analysis of drone implementation in agricultural practices through case studies.
Advantages of Drone-Aided Soil Analysis
Drones offer significant advantages over traditional soil sampling methods. Their ability to cover vast areas rapidly and efficiently makes them ideal for large agricultural fields. Drones can collect data in areas that are difficult or dangerous for human access, providing comprehensive coverage. The high-resolution imagery captured by drones enables detailed mapping of soil nutrient variations, providing a much more accurate picture of the field’s needs.
This allows for precise targeted nutrient application, saving resources and reducing environmental impact.
History of Drone Technology in Agriculture
The integration of drone technology in agriculture is relatively recent but has seen rapid advancements. Initial applications focused on aerial imagery for crop monitoring, but the technology quickly expanded to include soil analysis. Early adopters recognized the potential for improved efficiency and accuracy in managing soil nutrients. Ongoing research and development continue to push the boundaries of what drones can achieve in precision agriculture, leading to more sophisticated sensors and analysis techniques.
This ongoing evolution promises even greater accuracy and efficiency in the future.
Types of Drones Used for Soil Analysis
The selection of a drone for soil analysis depends on the specific needs of the agricultural operation. Factors such as the size of the field, the type of soil, and the desired level of detail all play a role. A range of drones, equipped with various sensors, are available to address these diverse needs.
Drone Type | Specifications | Capabilities |
---|---|---|
Lightweight, fixed-wing drones | Relatively inexpensive, long flight times, high-resolution imagery | Suitable for large field surveys, capturing broad-scale nutrient patterns |
Multirotor drones | Versatile, maneuverable, capable of close-range imagery | Excellent for detailed mapping of small areas, capturing fine-scale variations |
Hybrid drones | Combining features of fixed-wing and multirotor drones | Offering a balance of speed, maneuverability, and image resolution |
Drone-Based Soil Sensing Technologies
Drones are revolutionizing agricultural practices, and soil analysis is no exception. Equipped with advanced sensing technologies, drones can rapidly and efficiently collect data on soil properties, enabling farmers to optimize nutrient management and boost crop yields. This detailed analysis of drone-based soil sensing technologies will explore the various methods used, their underlying principles, and how they facilitate targeted nutrient management.The integration of advanced sensing technologies into drones allows for a comprehensive and cost-effective approach to soil analysis.
This capability is particularly valuable in large agricultural fields where traditional methods can be time-consuming and expensive. Furthermore, the ability to repeatedly monitor soil conditions over time provides valuable insights into dynamic changes in soil properties, aiding in the development of tailored management strategies.
Multispectral and Hyperspectral Sensing
Multispectral and hyperspectral sensors capture reflected light across different wavelengths, providing valuable information about the soil’s composition and properties. These technologies are instrumental in identifying nutrient deficiencies and other soil health indicators.Multispectral imaging utilizes a limited number of narrow spectral bands, while hyperspectral imaging captures a much broader range of wavelengths. This allows for a more detailed analysis of the soil’s chemical and physical characteristics.
The spectral reflectance patterns of different soil components are unique, allowing for the identification of various elements and compounds. For instance, variations in chlorophyll content can indicate nutrient deficiencies.
- Principle of Multispectral Sensing: Multispectral sensors measure reflected light in specific bands, each corresponding to a different color or wavelength. Variations in reflectance across these bands are indicative of different soil components and conditions. This approach provides a quick overview of soil properties.
- Principle of Hyperspectral Sensing: Hyperspectral sensors capture reflected light over a much wider range of wavelengths, providing a highly detailed spectral signature of the soil. The detailed information allows for the detection of subtle variations in soil composition, which can be used to identify nutrient deficiencies and other soil health issues.
- Nutrient Deficiency Detection: Different spectral bands are sensitive to different nutrients. For example, nitrogen deficiency can manifest as a change in the reflectance in the red edge region. Similarly, phosphorus deficiency can exhibit specific reflectance patterns in other spectral bands.
Thermal Sensing
Thermal sensors measure the emitted thermal radiation from the soil surface. This information is particularly useful for assessing soil temperature and moisture content. The relationship between thermal radiation and soil temperature allows for estimations of the heat flow within the soil. This can provide critical insights into water retention and drainage capacity. For example, areas with high thermal inertia often correlate with areas having a high water retention capacity.
Soil Moisture Content Measurement
Soil moisture content is a crucial factor in agricultural productivity. Drones equipped with specific sensors can precisely measure soil moisture levels, providing critical data for irrigation management. The different sensors employed utilize various principles to determine moisture levels.
- Microwave Sensors: These sensors operate at microwave frequencies, penetrating the soil surface to measure soil moisture. They are robust and provide reliable measurements even in varied soil conditions. The signal strength is affected by soil moisture content, allowing for the determination of moisture levels.
- Time Domain Reflectometry (TDR) Sensors: TDR sensors measure the time it takes for a signal to travel through the soil. The speed of the signal is directly related to the soil’s dielectric constant, which is influenced by the moisture content. These sensors provide accurate and timely measurements of soil moisture.
- Capacitive Sensors: These sensors use capacitance principles to measure the soil’s dielectric constant, which is directly related to the moisture content. These sensors are relatively inexpensive and provide good real-time readings. Their accuracy, however, can be influenced by the soil’s texture and other factors.
Comparison of Sensing Technologies
Sensing Technology | Strengths | Weaknesses |
---|---|---|
Multispectral | Relatively inexpensive, quick data acquisition, good overview of soil properties | Limited spectral resolution, less detailed information compared to hyperspectral |
Hyperspectral | High spectral resolution, detailed information about soil composition, allows for the identification of subtle differences | More expensive, data processing can be complex, may require more sophisticated algorithms |
Thermal | Provides insights into soil temperature and heat flow, valuable for assessing water retention | Measurements are affected by atmospheric conditions, data interpretation can be challenging |
Microwave/TDR/Capacitive | Precise measurements of soil moisture, crucial for irrigation management | Microwave sensors may require calibration for different soil types, TDR and capacitive sensors can be affected by soil texture |
Data Acquisition and Processing
Drone-aided soil analysis hinges on precise data collection and meticulous processing. Accurate soil nutrient mapping relies heavily on the quality of this initial stage. The procedures employed directly impact the reliability and usability of the resulting data for targeted nutrient management strategies.High-resolution imagery from drones, combined with spectral data, allows for detailed assessments of soil properties. Sophisticated algorithms analyze this data to identify patterns and variations in nutrient content across a field, providing crucial insights for optimized fertilizer application.
Drone-Based Soil Data Collection Procedures
Data acquisition involves careful planning and execution. Pilots utilize pre-programmed flight paths, often employing GPS-guided systems, to ensure consistent coverage of the target area. Drones equipped with multispectral or hyperspectral sensors capture images across a range of wavelengths, reflecting different soil characteristics. The optimal flight altitude and overlap between images are meticulously calculated to maximize spatial resolution and minimize data gaps.
Multiple flights might be necessary to achieve complete coverage of large fields. Weather conditions, including cloud cover and wind speed, significantly impact data quality and necessitate careful scheduling.
Data Pre-processing Techniques
Pre-processing steps are crucial for ensuring the accuracy and reliability of the data. Radiometric correction adjusts for variations in sensor response, such as intensity and light levels. This correction helps to eliminate artifacts and ensures that spectral values accurately reflect the soil properties. Atmospheric correction removes the influence of atmospheric components, which can distort the spectral signatures of the soil.
These corrections are essential for comparing data from different flights and seasons, and for ensuring consistent data quality. Various algorithms are employed for these corrections, ranging from simple linear models to more complex radiative transfer models.
Georeferencing and Data Alignment
Accurate georeferencing is critical to relate the collected data to specific locations within the field. This involves aligning the drone-acquired imagery with a known geographic coordinate system, often utilizing GPS data from the drone. Sophisticated image processing software and algorithms align multiple images to form a mosaic, ensuring seamless coverage across the entire study area. Precise alignment is paramount to ensure accurate spatial representation of the soil properties.
Misalignment can lead to errors in the mapping and interpretation of soil nutrient variations.
Raw Data Preparation for Analysis
Organizing and preparing the raw data for analysis is a vital step. Raw data files, such as images and sensor readings, are typically stored in specific formats (e.g., GeoTIFF) suitable for processing. Metadata, including flight parameters, sensor settings, and geographic coordinates, are meticulously recorded and attached to the data files. This ensures the traceability and reproducibility of the analysis.
Data quality checks are performed to identify and eliminate any anomalies or errors in the collected data, minimizing potential inaccuracies in the final analysis.
Data Acquisition and Processing Pipeline Flowchart
A flowchart illustrating the entire data acquisition and processing pipeline would show a linear progression from data collection to analysis. It would begin with pre-flight planning, followed by drone-based data acquisition, including image capture and GPS recording. Then, the data would move through pre-processing steps, including radiometric and atmospheric corrections. This would be followed by georeferencing and data alignment.
Finally, the pipeline would conclude with data organization and preparation for analysis.
Soil Nutrient Mapping and Analysis

Drone-collected data is revolutionizing agricultural practices by enabling precise and efficient soil nutrient management. This detailed analysis, encompassing various techniques and algorithms, allows farmers and researchers to create comprehensive maps illustrating soil nutrient concentrations, ultimately optimizing crop yields and minimizing environmental impact. The ability to visualize spatial variations in nutrients is critical for targeted fertilization, reducing unnecessary input costs, and enhancing sustainability.The accuracy of these maps depends heavily on sophisticated data processing and interpretation techniques.
This includes employing interpolation methods to predict nutrient levels in un-sampled areas, and leveraging machine learning to identify intricate patterns within the data. A crucial component is developing user-friendly dashboards that present these findings in an accessible and informative manner.
Methods for Generating Soil Nutrient Maps
Generating accurate maps of soil nutrient concentrations requires careful consideration of the drone-collected data. The spatial resolution of the imagery, the spectral characteristics of the soil, and the chosen analysis techniques all play significant roles in the final map’s reliability. Precise mapping relies on sophisticated algorithms that extract meaningful information from the data. These algorithms are often tailored to specific nutrient types, considering their unique absorption and reflection properties across different wavelengths.
For instance, algorithms for phosphorus detection might differ significantly from those designed for nitrogen.
Interpolation Techniques for Spatial Prediction
Interpolation is a crucial step in creating soil nutrient maps, enabling estimations of nutrient levels in areas where direct measurements are unavailable. Various methods exist, each with its strengths and weaknesses. Different interpolation techniques, such as inverse distance weighting, kriging, and spline interpolation, have varying degrees of accuracy depending on the spatial distribution of sample points and the characteristics of the soil.
Choosing the appropriate method hinges on the specific characteristics of the dataset and the desired level of accuracy.
Machine Learning for Identifying and Classifying Soil Nutrient Patterns
Machine learning algorithms are increasingly utilized to identify and classify soil nutrient patterns. These algorithms excel at recognizing complex relationships and subtle trends within large datasets, tasks that are often difficult for traditional methods. For example, support vector machines (SVM) or random forests can be trained on historical data to predict nutrient levels based on drone imagery and soil characteristics.
These methods often provide superior accuracy compared to traditional interpolation techniques, particularly in areas with complex or highly variable nutrient distributions.
Creating an Informative Dashboard
A well-designed dashboard is essential for effectively presenting the analyzed data. The dashboard should display soil nutrient maps in a user-friendly format, allowing for easy visualization and interpretation. Interactive tools, enabling zooming and filtering, should be included to facilitate detailed examination of specific regions. Key parameters, such as nutrient concentrations and associated uncertainty, should be clearly labeled.
A dashboard that allows overlaying of the nutrient maps with existing farm data or satellite imagery enhances its usability.
Comparison of Interpolation Methods
Interpolation Method | Description | Suitability for Different Soil Types | Advantages | Disadvantages |
---|---|---|---|---|
Inverse Distance Weighting (IDW) | Weights sample points based on their proximity to the prediction location. | Relatively straightforward, suitable for relatively homogeneous soil types. | Computationally efficient. | Accuracy can be affected by irregularly spaced sample points. |
Kriging | Accounts for spatial autocorrelation in the data. | Effective for heterogeneous soil types with significant spatial variability. | High accuracy. | More computationally intensive than IDW. |
Spline Interpolation | Fits a smooth curve or surface to the sample points. | Suitable for complex soil types and irregular spatial patterns. | Produces smooth results. | Can be sensitive to outliers. |
Targeted Nutrient Management Strategies
Precision agriculture, driven by drone-aided soil analysis, is revolutionizing farming practices. By creating detailed maps of soil nutrient content, farmers can now tailor fertilization strategies to specific areas within a field, optimizing nutrient uptake and minimizing waste. This targeted approach leads to increased crop yields, reduced environmental impact, and enhanced farm profitability.
Developing Targeted Nutrient Management Plans
Drone-generated soil maps provide a granular understanding of nutrient distribution across a field. This data allows for the development of customized nutrient management plans, addressing the specific needs of each area. Farmers can identify areas deficient in particular nutrients and apply fertilizers accordingly, avoiding unnecessary application in areas with adequate levels. This targeted approach maximizes fertilizer use efficiency and reduces environmental pollution.
Fertilization Strategies for Optimized Nutrient Uptake
Different fertilization strategies can be employed to optimize nutrient uptake and minimize waste. These strategies often involve applying fertilizers in multiple smaller doses rather than one large application. This allows the roots to absorb nutrients more effectively, reducing leaching losses and promoting healthier plant growth. Variable-rate application, a technique facilitated by drone data, is crucial in this regard.
Tailoring Fertilization Plans for Specific Field Areas
Drone-generated maps allow for a precise analysis of nutrient deficiencies in specific field areas. This detailed information enables farmers to tailor fertilization plans to meet the unique needs of each area. For example, a section of a field exhibiting low nitrogen levels could receive a targeted application of nitrogen fertilizer, while areas with adequate nitrogen levels would receive minimal or no application.
This precision avoids over-fertilization, reducing costs and environmental impact.
Precision Agriculture’s Role in Enhancing Farm Efficiency and Sustainability
Precision agriculture, with its reliance on drone-aided soil analysis, plays a pivotal role in enhancing farm efficiency and sustainability. By optimizing fertilizer use and minimizing waste, farmers can reduce production costs, improve crop yields, and decrease their environmental footprint. This approach promotes a more sustainable agricultural system, aligning with global efforts to address food security and environmental concerns.
Implementing a Cost-Benefit Analysis for Drone Technology
Implementing a cost-benefit analysis for drone-aided soil analysis requires careful consideration of both initial and ongoing costs. The initial investment in drones, software, and associated equipment must be weighed against the potential savings in fertilizer costs, labor costs, and potential yield increases. For instance, a farmer might calculate the return on investment (ROI) based on the estimated savings in fertilizer expenses and the potential increase in crop yields in a specific area, considering the expected duration of drone use and its impact on the overall farming process.
Real-world case studies of farms that have adopted this technology can provide valuable data for assessing the ROI and cost-effectiveness of the investment. Furthermore, the long-term sustainability benefits, such as reduced environmental impact and enhanced resource management, are also key considerations in the analysis.
Challenges and Future Directions
Precision agriculture, relying increasingly on drone technology, faces hurdles in soil analysis. While drones offer significant potential for targeted nutrient management, overcoming limitations in accuracy, cost-effectiveness, and regulatory frameworks is crucial for widespread adoption. The environmental impact of drone use, too, must be carefully considered. This section explores these challenges and potential future advancements.
Potential Challenges in Drone-Aided Soil Analysis
Several challenges hinder the widespread adoption of drone-aided soil analysis for precision agriculture. These range from technical limitations in data acquisition and processing to regulatory complexities and environmental considerations. Addressing these issues is essential for maximizing the technology’s benefits.
- Data Acquisition and Processing Challenges: Varied soil types, terrain complexities, and weather conditions can significantly impact the accuracy and reliability of drone-acquired data. Variations in soil moisture, vegetation cover, and lighting conditions can introduce noise and artifacts in the sensor data. Developing robust data processing algorithms to account for these factors is crucial to improving the accuracy of soil nutrient mapping.
For example, sophisticated image processing techniques, such as employing deep learning algorithms, can enhance the extraction of relevant information from drone-acquired imagery, improving the overall accuracy of soil nutrient analysis.
- Cost-Effectiveness and Accessibility: The initial investment in drone hardware, software, and skilled personnel can be prohibitive for many small-scale farmers. The cost of data processing and interpretation also needs to be considered. To improve accessibility, innovative business models, such as shared drone platforms or subsidized data processing services, may be needed. This would enable more farmers to access this technology and benefit from its precision.
- Accuracy and Validation: The accuracy of drone-derived soil nutrient maps needs to be validated against traditional methods of soil sampling. Establishing robust validation procedures, including extensive field trials, is vital to ensuring the reliability and trustworthiness of the data. The comparison of drone-derived maps with ground-truth data collected using traditional methods, such as soil pits and laboratory analyses, is essential for establishing the confidence intervals of the drone-based estimations.
This comparative analysis would reveal the limitations of the drone technology and the extent of the discrepancies between drone-derived and ground-truth maps.
Limitations of Drone Technology and Potential Solutions
While drone technology offers a promising approach to soil analysis, inherent limitations must be addressed to ensure its efficacy. Solutions for these limitations are being explored by researchers and agricultural professionals.
- Limited Spatial Resolution: Current drone sensors may not have the resolution needed for highly localized or detailed soil analysis. For example, in areas with heterogeneous soil types or where very precise nutrient management is required, the current resolution might not provide adequate detail. Future advancements in sensor technology, including the development of high-resolution multispectral and hyperspectral sensors, are expected to enhance the spatial resolution of drone-derived soil maps.
The development of multi-sensor platforms, which combine multiple types of sensors on a single drone, may also provide comprehensive data for more accurate analysis.
- Environmental Impact of Drone Use in Agriculture: The environmental impact of drone use in agriculture, including noise pollution, energy consumption, and potential pesticide exposure, needs careful consideration. Strategies to minimize these impacts, such as optimizing drone flight paths and utilizing energy-efficient drone models, are crucial for sustainable agriculture. The implementation of stricter regulations regarding drone operation, including limitations on flight altitudes and noise levels, can further reduce environmental concerns.
Future Research Directions
Continued research and development are essential to enhance the capabilities of drone-aided soil analysis. This includes exploring new sensor technologies, data processing algorithms, and validation methodologies.
- Advancements in Drone Technology: Further advancements in drone technology, such as miniaturization, enhanced sensor capabilities, and improved battery life, will improve the accessibility and effectiveness of drone-based soil analysis. For example, the integration of autonomous navigation systems with drone technology will optimize flight paths and reduce human intervention.
- Integration with Other Technologies: The integration of drone-based soil analysis with other precision agriculture technologies, such as variable rate application systems and soil moisture sensors, can lead to more efficient and targeted nutrient management. For example, the combination of drone-derived soil nutrient maps with real-time soil moisture data can provide farmers with more accurate information for irrigation and fertilization decisions.
Legal and Regulatory Considerations
The use of drones in agriculture necessitates careful consideration of legal and regulatory frameworks.
Aspect | Considerations |
---|---|
Federal Regulations | Federal regulations governing drone operations, including airspace restrictions and safety standards, need to be adhered to. Compliance with these regulations is crucial to avoid penalties and ensure safe drone operation. |
State and Local Regulations | State and local regulations may impose additional requirements on drone operations, including permitting requirements and flight restrictions within specific areas. |
Data Privacy and Security | Data privacy and security considerations are critical when using drone-acquired data. Protecting the confidentiality of farmer data and ensuring the secure storage and transmission of data are essential. |
Liability and Insurance | Liability and insurance policies should be in place to cover potential damages or accidents related to drone operations. |
Case Studies and Applications
Drone-aided soil analysis is rapidly transforming agricultural practices, offering precision and efficiency in nutrient management. This technology promises to optimize fertilizer application, leading to increased yields and reduced environmental impact. Successful implementations demonstrate the potential for widespread adoption, boosting profitability and sustainability in farming.
Successful Applications in Diverse Cropping Systems
Real-world applications of drone-aided soil analysis showcase its versatility across various cropping systems. Different crops have varying nutrient requirements, and this technology allows for tailored management strategies. The flexibility of drones in adapting to different terrains and crop types is a key factor in its success.
- Precision Agriculture in Wheat Fields: A case study in the Canadian prairies demonstrates significant yield increases in wheat fields using drone-based soil mapping. By identifying areas with nutrient deficiencies, farmers were able to apply fertilizers precisely, resulting in a 15% increase in wheat yields compared to traditional methods. The technology helped minimize fertilizer overuse, thereby reducing environmental pollution and increasing economic returns.
- Targeted Nutrient Management in Rice Paddies: In Southeast Asian rice paddies, drones were employed to analyze soil nutrient levels in different parts of a rice field. This analysis allowed for precise application of nitrogen and phosphorus fertilizers, reducing overall fertilizer usage by 20% and improving rice yield while decreasing pollution in the water systems. This targeted approach improved the overall sustainability of the agricultural process.
- Optimizing Fruit Orchard Productivity: Orchard owners in California have implemented drone-based soil analysis to manage nutrient deficiencies in their fruit trees. The detailed mapping of soil nutrients facilitated precise fertilization, resulting in improved fruit quality and quantity, and a substantial increase in yield. This precise approach allowed for optimized nutrient levels for the specific varieties of fruit, leading to a more profitable orchard operation.
Challenges and Mitigation Strategies
While the potential of drone-aided soil analysis is substantial, challenges exist. Cost-effectiveness and data interpretation remain key concerns. However, advancements in technology and increasing data accessibility are gradually overcoming these obstacles.
- Cost of Equipment and Data Analysis: The initial investment in drone technology and software for data processing can be substantial. However, the long-term benefits in terms of increased yield and reduced input costs can offset this investment. Further, the emergence of cloud-based data analysis platforms is reducing the financial burden of processing large datasets.
- Data Interpretation and Variability: Accurate interpretation of drone-acquired data requires skilled personnel and sophisticated software. The variability in soil properties and crop types necessitates a tailored approach to data interpretation, which is a significant but surmountable challenge. Further, data validation procedures and comparisons to traditional methods are necessary to ensure reliability.
- Addressing Regional Variations: Different soil types and environmental conditions necessitate adjustments in data interpretation and analysis procedures. For instance, in arid regions, soil moisture content needs to be factored into the nutrient analysis. This is achieved through calibration and refinement of algorithms tailored to specific geographic conditions.
Impact on Yield and Profitability
The positive impact of drone-aided soil analysis on agricultural yield and profitability is substantial. The precision and efficiency of targeted nutrient management directly translate into higher yields and reduced input costs.
“Studies have shown a demonstrable increase in crop yields of 10-20% through the implementation of drone-aided soil analysis.”
Reduced fertilizer use and optimized nutrient application translate into significant cost savings for farmers. These benefits contribute to a more sustainable and profitable agricultural sector.
Wrap-Up
In conclusion, drone-aided soil analysis for targeted nutrient management represents a significant advancement in precision agriculture. By combining advanced sensing technologies with data processing and analysis, this approach offers a more sustainable and profitable farming model. The future of agriculture likely lies in this integration of technology and agricultural practices, promising greater efficiency and resource conservation.