Drone technology is rapidly transforming agriculture, offering a precise and efficient approach to pest management. This new method, drone-based crop monitoring, leverages aerial imagery and advanced data analysis to detect and control pests, potentially revolutionizing agricultural practices. Traditional methods often suffer from limitations in coverage and accuracy, while drone technology offers a more comprehensive and timely solution.
This comprehensive analysis explores the intricacies of drone-based crop monitoring for pest detection and control, from the different drone types and image acquisition methods to the sophisticated algorithms and control strategies employed. The detailed examination also encompasses data analysis, potential challenges, and future advancements, offering a comprehensive understanding of this emerging technology in agriculture.
Introduction to Drone-Based Crop Monitoring
Unmanned aerial vehicles (UAVs), commonly known as drones, are rapidly transforming agricultural practices. Their versatile capabilities, combined with advanced sensor technology, provide farmers with unprecedented insights into their crops, enabling proactive pest management and optimized resource allocation. This innovative approach promises to increase yields, reduce costs, and enhance sustainability in farming.Drones equipped with high-resolution cameras and specialized sensors can meticulously survey vast tracts of land, capturing detailed images and data that traditional methods often miss.
This detailed information allows for the early detection of pest infestations, enabling timely interventions to prevent widespread damage and economic losses.
Drone Technology in Agriculture
Drone technology is revolutionizing agriculture by providing a comprehensive approach to crop monitoring. Employing aerial imagery and sensor data, drones can assess crop health, identify potential problems, and guide targeted interventions. This capability offers a significant advancement over traditional methods, which often rely on ground-based inspections, resulting in delayed detection and inefficient resource allocation.
Role of Drones in Pest Detection and Control
Drones equipped with advanced image processing software can identify subtle signs of pest infestations. These signs, often undetectable by the human eye, can be picked up by the drones’ high-resolution cameras and analyzed to pinpoint the location and severity of the infestation. This data allows farmers to apply pesticides precisely to the affected areas, minimizing environmental impact and maximizing the effectiveness of the treatment.
Benefits of Drone-Based Monitoring Compared to Traditional Methods
The use of drones for pest detection and control offers several significant advantages over traditional methods. Drones provide a more comprehensive view of the entire field, allowing for rapid and wide-scale assessments. This, in turn, translates to earlier detection of pest problems, enabling faster and more targeted responses. The ability to monitor large areas with minimal human intervention reduces labor costs and ensures coverage of remote or difficult-to-access regions.
Comparison of Drone-Based and Traditional Pest Detection Methods
Method | Cost | Time | Accuracy |
---|---|---|---|
Drone-Based | Higher initial investment for drone and software, but lower ongoing costs compared to extensive ground surveys. Variable costs depending on flight time and data analysis needs. | Rapid assessment of large areas, enabling early intervention. Typically quicker than traditional methods. | High accuracy due to detailed imagery and advanced image processing software. Potential for more precise targeting of pest infestations. |
Traditional Methods (e.g., ground surveys) | Lower initial investment, but significantly higher ongoing costs associated with extensive labor and potential for delayed intervention. | Slower assessment of areas, often resulting in delayed intervention and potential for widespread pest damage. | Lower accuracy due to limited field of view and potential for human error. May miss subtle signs of pest infestations. |
Types of Drones Used for Crop Monitoring
Advancements in drone technology are revolutionizing agricultural practices, particularly in pest detection and control. Drones offer a cost-effective and efficient alternative to traditional methods, enabling farmers to monitor vast areas rapidly and precisely. This detailed analysis explores the diverse types of drones employed in crop monitoring, highlighting their specific capabilities and limitations.
Different Drone Types Suitable for Crop Monitoring
Various drone types cater to specific agricultural needs. The choice of drone depends on factors like the size of the field, the type of crops, and the desired level of detail for pest detection. Different drone models are optimized for diverse tasks and environmental conditions, contributing to a multifaceted approach to crop monitoring.
- Fixed-wing drones, characterized by their aerodynamic design and high speed, are particularly well-suited for extensive coverage of large agricultural fields. Their ability to fly at high altitudes and maintain stable flight paths allows for efficient data acquisition over wide areas. This efficiency is especially advantageous for early detection of pest infestations, allowing timely intervention.
- Multirotor drones, often referred to as quadcopters, are highly maneuverable and capable of hovering. Their versatility makes them suitable for detailed inspections of smaller fields and precise identification of pest outbreaks. Their agility allows for close-up observations, enabling more detailed analyses of plant health and damage.
- Hybrid drones, combining features of both fixed-wing and multirotor drones, offer a blend of high-speed coverage and detailed inspection capabilities. This hybrid approach can significantly enhance the efficiency of pest detection, especially when large areas need comprehensive monitoring, followed by targeted investigations in specific problem areas.
Comparative Analysis of Drone Models for Pest Detection
Different drone models exhibit varying capabilities for pest detection. Key factors influencing the selection include payload capacity, sensor integration, flight time, and data processing capabilities.
Drone Type | Features | Advantages | Limitations |
---|---|---|---|
Fixed-wing drones | High speed, long flight times, large-area coverage, often equipped with high-resolution cameras | Efficient for large-scale monitoring, timely detection of widespread infestations | Limited maneuverability, less suitable for detailed inspections, potential for higher operational costs |
Multirotor drones | High maneuverability, precise hovering, ability to carry diverse sensors, capable of close-up inspections | Detailed inspections, optimal for identifying localized pest outbreaks, good for precision agriculture | Shorter flight times, smaller coverage area, potential for reduced speed compared to fixed-wing drones |
Hybrid drones | Combines speed and maneuverability of both fixed-wing and multirotor, higher payload capacity compared to multirotor | Efficient for large-scale monitoring with detailed inspection of problem areas | More complex operation and maintenance, costlier than multirotor models |
Technical Specifications of Drones for Agricultural Applications
Agricultural drones often incorporate advanced sensors and imaging technologies to identify pest infestations. Key technical specifications include camera resolution, sensor types, and data processing capabilities. For example, thermal imaging cameras can detect subtle temperature differences in plants indicative of pest presence.
Obtain a comprehensive document about the application of drone technology for livestock management and monitoring that is effective.
High-resolution multispectral cameras are increasingly used to analyze the spectral reflectance of crops, helping identify stress indicators that might be missed by the naked eye, enabling early detection of pest-related issues.
These technical specifications contribute to the accuracy and efficiency of pest detection and control strategies.
Image and Data Acquisition Methods

Drone-based crop monitoring is rapidly evolving as a crucial tool for precise pest detection and targeted control. This advanced approach leverages the power of aerial imagery and sophisticated data analysis to identify pest infestations early, minimizing crop damage and maximizing yields. The methods employed for image and data acquisition play a critical role in the effectiveness of this technology.The process of acquiring high-quality images and data from drones is a multi-faceted procedure.
Different sensors and image processing techniques are employed to identify and classify pests accurately. This precision is vital for effective pest management strategies.
Image Acquisition Process Using Drones
Drone-based image acquisition involves the controlled flight of drones equipped with high-resolution cameras and sensors. The drones meticulously map the crop fields, capturing detailed images from various angles. The optimal flight paths and altitudes are pre-programmed to ensure comprehensive coverage of the field. This allows for a comprehensive assessment of the entire area, rather than relying on localized observations.
Sensors Used for Data Capture
Various sensors are employed for acquiring data, including multispectral and hyperspectral cameras. Multispectral cameras capture images in multiple narrow bands of the electromagnetic spectrum, while hyperspectral cameras capture a significantly larger number of bands. These sensors provide a detailed representation of the crop’s spectral signature, which is crucial for detecting subtle variations indicative of pest infestations.Furthermore, thermal imaging cameras are increasingly used to identify stress in plants, a possible symptom of pest infestation.
This allows for early detection of problems that might otherwise go unnoticed.
Image Processing Techniques for Pest Identification
Sophisticated image processing techniques are applied to the acquired drone imagery. These techniques involve algorithms that identify patterns, anomalies, and subtle changes in the crop’s appearance that could indicate pest presence. Image segmentation, for example, separates the crop from the background, enabling focused analysis of the crop itself. Other techniques include feature extraction, which identifies key characteristics of the plants or pests, and classification algorithms that categorize these features to identify specific pests.
Flowchart of Data Acquisition from Drone Imagery
The following flowchart illustrates the process of data acquisition:
+-----------------+ | Start | +-----------------+ | Drone Flight | +-----------------+ | Image Acquisition | +-----------------+ | Data Transmission| +-----------------+ | Image Processing | +-----------------+ | Pest Detection | +-----------------+ | Analysis | +-----------------+ | Report Generation | +-----------------+ | End | +-----------------+
This flowchart provides a concise overview of the entire process, from the initial flight to the final report generation.
Utilization of Spectral Bands for Pest Identification
Different spectral bands provide unique insights into the health and condition of the crops. For instance, red edge reflectance, a characteristic feature of vegetation, can be used to identify stress or damage caused by pests. Near-infrared (NIR) reflectance can be crucial for detecting subtle changes in the crop canopy that might indicate the presence of pests or diseases.
Different pests exhibit different spectral signatures. This allows for the development of algorithms that identify specific spectral signatures associated with particular pests, facilitating precise and targeted pest control strategies. For example, if a specific pest exhibits a particular reflectance in the red edge, a drone equipped with a sensor capable of detecting this characteristic can provide a prompt identification, enabling timely action.
Pest Detection Algorithms and Techniques
Drone-based crop monitoring is rapidly evolving, with sophisticated algorithms playing a crucial role in identifying and quantifying pest infestations. These algorithms, often powered by machine learning, analyze the imagery captured by drones to pinpoint areas of concern, allowing for targeted interventions and minimizing pesticide use. Accurate pest detection is essential for sustainable agriculture and maximizing crop yields.
Machine Learning Algorithms for Pest Detection
Machine learning algorithms are transforming pest detection in agriculture. These algorithms are trained on vast datasets of images and associated pest information to learn patterns and characteristics indicative of pest presence. Commonly used algorithms include Convolutional Neural Networks (CNNs), Support Vector Machines (SVMs), and Random Forest algorithms. CNNs excel at identifying complex patterns in images, making them particularly effective in recognizing subtle visual cues of pests.
SVMs are effective in classifying images based on learned features, while Random Forests provide robust predictions by averaging the results of multiple decision trees. These algorithms, when combined with advanced image processing techniques, provide a powerful tool for precise and timely pest identification.
Steps in Applying Algorithms to Drone Imagery
The process of applying these algorithms to drone imagery involves several crucial steps. First, the drone captures high-resolution images of the crops. Second, the images are preprocessed to enhance the quality of the data, potentially through techniques such as noise reduction and contrast adjustments. Third, the preprocessed images are fed into the chosen machine learning algorithm, which learns to identify the characteristics of the pest.
Fourth, the trained algorithm is then used to analyze new images, identifying areas where pests are present. Finally, the output is often visualized as a map or heatmap, highlighting the regions with high pest density, allowing for targeted intervention.
Role of Image Processing Techniques
Image processing techniques are essential for improving the accuracy and efficiency of pest detection. Techniques like image segmentation, feature extraction, and object detection are employed to isolate pests from the background and identify their key features. Image segmentation divides the image into different regions based on color, texture, or shape, isolating the pest from the surrounding crop. Feature extraction identifies distinctive characteristics of the pests, such as size, shape, or color patterns, enabling the algorithm to differentiate them from other objects.
Object detection locates and classifies the pest within the image, providing accurate localization and identification. These techniques, combined with machine learning, are crucial for achieving reliable pest detection.
Comparison of Pest Detection Algorithms
Different machine learning algorithms have varying strengths and weaknesses in pest detection. CNNs are particularly effective in identifying complex patterns, but require significant training data. SVMs are relatively efficient in classifying images, but may struggle with nuanced patterns. Random Forests offer robustness but might not achieve the same level of accuracy as CNNs. Choosing the optimal algorithm depends on factors such as the type of pest, the quality of the drone imagery, and the available computational resources.
Table of Algorithms and Their Characteristics
Algorithm | Strengths | Weaknesses | Applications |
---|---|---|---|
Convolutional Neural Networks (CNNs) | Excellent at identifying complex patterns, high accuracy in image recognition. | Requires large amounts of training data, computationally intensive. | Identifying various pests in diverse environments, detecting subtle differences in pest characteristics. |
Support Vector Machines (SVMs) | Efficient classification, relatively less computationally intensive. | May struggle with complex patterns, less effective for detailed pest recognition. | Initial screening for pest presence, quick assessment of large areas. |
Random Forest | Robust predictions, less susceptible to outliers, good for diverse pest types. | May not achieve the same level of accuracy as CNNs, performance can vary depending on the dataset. | General pest detection in various scenarios, including mixed pest infestations. |
Control Strategies and Implementation
Precision agriculture is rapidly integrating drone technology for targeted pest control. This allows farmers to apply pesticides and other control agents with unprecedented accuracy, minimizing environmental impact and maximizing crop yield. Drones equipped with advanced sensors and application systems are revolutionizing how we approach pest management.
Targeted Pest Control Using Drone Data
Drone-based crop monitoring provides detailed spatial data on pest infestations. Analyzing this data, farmers can identify areas with high pest densities and tailor treatment strategies accordingly. This targeted approach significantly reduces the amount of pesticide used compared to blanket spraying, minimizing potential harm to beneficial insects and the environment. By identifying and isolating the problem areas, drone technology allows for more efficient and sustainable pest management.
Drone-Based Pesticide Application
Drones equipped with specialized spray systems are now capable of applying pesticides directly to affected areas. These systems are designed for precise application, ensuring that the pesticide reaches the target pests without contaminating healthy plants or the surrounding environment. This technology has the potential to reduce pesticide usage and associated costs while ensuring effective pest control. Precise targeting, minimized drift, and reduced overall chemical use are key benefits.
Example of a Comprehensive Pest Control Strategy
A comprehensive strategy often combines drone-based monitoring with other pest control methods. For example, in a field showing signs of aphids, drone imagery would identify the specific sections of the crop with high aphid concentrations. This data would then be used to guide the application of targeted pesticide spraying by the drone. Furthermore, monitoring can be used to assess the effectiveness of the pesticide and make necessary adjustments.
Procedures for Implementing Drone-Based Pest Control
Implementing drone-based pest control strategies in various agricultural settings requires careful planning and execution. Procedures vary based on the crop type, pest species, and the specific needs of the farm. Essential steps typically include:
- Pre-flight Assessment: This involves analyzing historical data on pest infestations, weather forecasts, and crop health. Drone pilots must also evaluate the flight plan to ensure optimal coverage of the affected areas.
- Data Acquisition: Drones equipped with high-resolution cameras and sensors capture images and data of the crops. The data collected must be accurate and representative of the pest distribution.
- Data Processing: The acquired data is processed using specialized software to identify pest infestations, assess their severity, and determine the optimal treatment areas.
- Targeted Application: Drones equipped with pesticide sprayers apply the chosen control agent to the designated areas. Calibration and precise spraying are critical to ensure the pesticide reaches the target pests effectively and minimizes drift.
- Post-treatment Monitoring: Following pesticide application, drones continue monitoring the treated areas to assess the effectiveness of the intervention and make necessary adjustments.
Flowchart for Drone-Based Pest Control Strategies
The following flowchart illustrates the steps involved in a typical drone-based pest control strategy:
“`
[Diagram of a flowchart. The flowchart would visually represent the steps listed above in a logical sequence. It would start with “Pre-flight Assessment” and end with “Post-treatment Monitoring”. Each step would be a box or oval, and arrows would connect the steps, showing the progression. The flowchart should be easily understood and visually appealing, with clear labels for each step.]
“`
Data Analysis and Interpretation
Drone-based crop monitoring is revolutionizing agriculture, offering unprecedented insights into crop health and potential threats. Crucially, the wealth of data collected requires sophisticated analysis to translate raw imagery into actionable strategies for pest management. Effective interpretation of this data is paramount for informed decision-making, enabling farmers to proactively address issues and optimize yield.
Data Processing and Analysis
The process of analyzing drone-collected data involves several crucial steps. First, the raw imagery is processed to remove noise and enhance image quality. This often involves calibration to ensure accurate measurements and georeferencing to establish the spatial context of the data. Next, image segmentation isolates specific areas of interest, such as individual plants or affected regions. Sophisticated algorithms then identify patterns indicative of pest infestations, stress, or other anomalies.
Quantitative analysis, including measurements of leaf area, biomass, and chlorophyll content, provides further insights into the severity of the problem.
Significance of Data for Decision-Making
The data generated by drone-based monitoring provides valuable information for proactive pest management. Detailed assessments of crop health, combined with pest presence and density information, allow farmers to pinpoint the location and extent of the problem. This precision is vital for targeted interventions, minimizing environmental impact and maximizing resource efficiency. By detecting pest infestations early, farmers can initiate timely control measures, preventing widespread damage and maintaining crop yield.
Data Visualization Techniques
Visual representation of the data is essential for effective analysis and communication. Color-coded maps depicting pest infestation levels allow for a clear overview of the affected areas. 3D models of the crop canopy, generated from the drone imagery, reveal spatial relationships and the distribution of pests within the field. Furthermore, interactive dashboards provide an integrated view of various data points, such as pest density, crop health, and environmental factors, streamlining the decision-making process.
Heatmaps, for instance, can highlight areas with a high concentration of pest activity.
Accuracy and Reliability in Data Interpretation
Accuracy and reliability are paramount in data interpretation. The precision of the drone’s flight path, the quality of the sensors, and the calibration of the imagery all influence the accuracy of the data. Sophisticated algorithms and rigorous validation procedures are essential to minimize errors. A critical step involves cross-referencing the drone data with ground-level observations and historical data to verify the findings.
Relationship Between Data Points and Pest Infestations
Data Point | Interpretation | Action | Impact |
---|---|---|---|
Reduced chlorophyll content in specific areas | Indicates stress or potential pest infestation, potentially caused by feeding or disease. | Investigate the area further, collect ground samples, and consider insecticide treatments in the identified area. | Early intervention prevents the spread of pests, reduces yield loss, and protects the entire crop. |
Increased presence of pest insects in a field segment | High pest density warrants immediate action. | Implement targeted insecticide applications in the affected area, potentially using precision spraying techniques. | Reduced pest population minimizes damage, maximizing yield and profit. |
Unusual leaf patterns (e.g., curling, discoloration) | Suggests a possible pest infestation or environmental stress. | Conduct a visual inspection of the affected area, compare to historical data, and potentially sample leaves for lab analysis. | Preventing the disease’s spread ensures crop health and prevents further loss. |
High moisture content in a specific area | Could indicate fungal diseases or pests that thrive in moist conditions. | Assess for potential fungal or pest issues, adjust irrigation schedules, or implement fungicide treatments if necessary. | Ensuring proper moisture management minimizes disease risk and enhances crop health. |
Challenges and Future Directions
Drone-based crop monitoring, while offering promising solutions for pest detection and control, faces hurdles in practical implementation. Economic viability, environmental impact, and ethical considerations need careful evaluation to ensure widespread adoption. The technology’s limitations in specific scenarios and potential future advancements are also key areas of investigation.
Potential Limitations of Drone-Based Pest Detection
Accurate pest detection relies on several factors. Drone-based systems can be affected by weather conditions, such as heavy rain or strong winds, which can obscure images and interfere with data acquisition. Furthermore, the resolution of the images captured by drones can be insufficient to identify subtle pest infestations, particularly in the early stages of an outbreak. Complex crop canopies and dense vegetation can also impede the drone’s ability to fully visualize the crop, leading to missed or inaccurate detections.
Finally, the algorithms used for pest identification need to be continually refined to adapt to different crop types, pest species, and environmental variations. For example, some pest species may exhibit camouflage or behavior patterns that make detection difficult.
Environmental Impact of Drone Use in Agriculture
The environmental impact of drone usage in agriculture needs careful consideration. While drones offer precision agriculture, their operation consumes energy, contributing to carbon emissions. Battery life, flight time, and the manufacturing process of drones need to be addressed to reduce their environmental footprint. Furthermore, the use of pesticides, though often necessary in conjunction with drone-based monitoring, can have detrimental effects on non-target organisms.
Drone operators must ensure careful application and adherence to environmentally responsible practices. The noise generated by drones can also impact the local ecosystem, potentially affecting pollinators and other wildlife.
Economic Feasibility of Drone Deployment
The economic viability of drone technology in agriculture hinges on several factors. The initial investment cost for drones, sensors, and data analysis software can be substantial. Operating costs, including maintenance, pilot training, and potential downtime, also need careful consideration. The return on investment (ROI) depends on factors such as the size of the farm, the prevalence of pests, and the effectiveness of the pest control strategies implemented.
Implementing drone technology in small-scale farms may not be economically viable, but for large-scale operations, it can prove cost-effective, especially when considering the potential savings from reduced pesticide use and increased yields. For example, in regions with high pest pressure, the reduced need for broad-spectrum pesticides can offset the initial investment costs.
Future Advancements in Drone Technology
Future advancements in drone technology promise to enhance crop monitoring capabilities. Developments in autonomous flight systems, improved sensor technology, and enhanced image processing algorithms will allow for more efficient and precise data acquisition. Increased battery life and longer flight times will also improve operational efficiency. For example, drones equipped with advanced multispectral cameras could identify subtle stress indicators in plants, enabling proactive intervention before pests cause significant damage.
The integration of AI and machine learning will further refine pest detection algorithms, improving accuracy and reducing the need for human intervention.
Ethical Considerations Associated with Drone Usage
Ethical considerations surrounding drone use in agriculture need careful consideration. Privacy concerns related to the collection and use of data from farms must be addressed. Regulations and protocols for data security and ownership are crucial. Drone operations must also adhere to existing airspace regulations, ensuring that the use of drones does not interfere with other aircraft or pose risks to public safety.
Transparency and accountability in the use of drone technology in agriculture are paramount to building trust and ensuring responsible innovation. For instance, clear guidelines for data sharing and access should be established to ensure that the benefits of drone technology are shared equitably.
Case Studies and Real-World Examples

Drone-based crop monitoring is rapidly transforming agricultural practices, offering a powerful tool for pest detection and targeted control. Successful implementations are demonstrating the technology’s potential to enhance yields, reduce pesticide use, and boost profitability across diverse agricultural landscapes. These case studies highlight the efficacy of drones in different crop types, revealing how real-world applications are impacting farming strategies.
Successful Implementations of Drone-Based Pest Monitoring
Numerous farms are successfully integrating drone technology for proactive pest management. Early detection, enabled by aerial imagery, allows for timely interventions, minimizing crop damage and maximizing yields. Drones equipped with advanced sensors provide detailed information on pest infestations, allowing for targeted pesticide application. This targeted approach reduces environmental impact compared to blanket spraying.
Effectiveness of Drones in Different Crop Types
The effectiveness of drone-based pest monitoring varies slightly depending on the crop type. For instance, row crops like soybeans and corn benefit from the ability of drones to rapidly survey large areas, enabling the detection of localized pest outbreaks. Fruit orchards and vineyards, with their intricate structures and dense canopies, may require specialized drone configurations and image analysis techniques for accurate pest identification.
Visual inspection combined with drone-captured data offers a more holistic approach to pest management.
Impact on Yield and Profitability
Drone data significantly influences yield and profitability. Early pest detection allows for timely interventions, preventing widespread infestations and substantial yield losses. This translates into direct economic benefits. Reduced pesticide use, a consequence of precise targeting, also lowers operational costs and minimizes environmental impact. Furthermore, the detailed data gathered by drones can be used to optimize irrigation and fertilization schedules, leading to further improvements in crop health and profitability.
Real-World Application: A Case Study in Soybean Production
“In a recent study of soybean farms, drone-based monitoring revealed early signs of a spider mite infestation in a specific field. The detailed imagery allowed for a precise identification of the affected areas. Subsequently, targeted pesticide application, guided by drone data, effectively controlled the infestation. The resulting yield increase was approximately 15%, leading to a significant improvement in overall farm profitability.”
Concluding Remarks
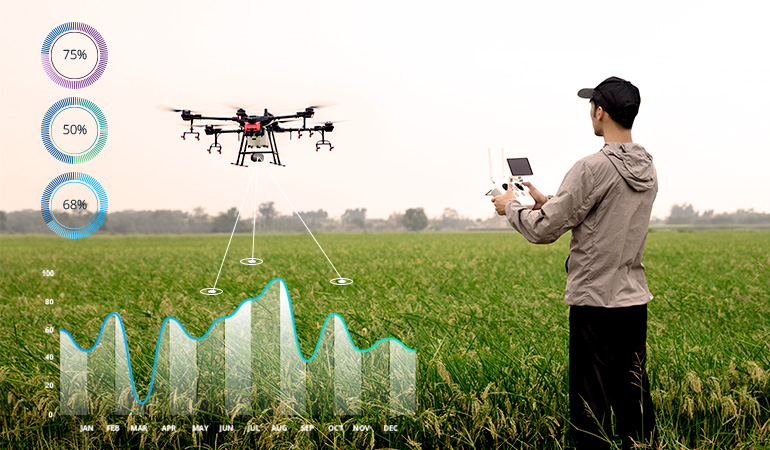
Drone-based crop monitoring for pest detection and control promises a significant leap forward in agricultural practices. By combining advanced technology with data analysis, farmers can achieve greater accuracy and efficiency in pest management, ultimately leading to improved crop yields and reduced reliance on harmful pesticides. The future of agriculture may well depend on the continued development and adoption of these innovative drone-based solutions.
1 Comment